with Dr. Mitchell Sipus
CalypsoAI Director of Product
Not so long ago, any deployment of machine learning (ML) within the government was an achievement, but recent trends have seen the widespread adoption of ML as a subset of applied data science. In recent years, federal agencies such as the DoD Joint AI Center (JAIC) and Government Services Administration (GSA) have introduced a range of programs to accelerate the adoption of artificial intelligence (AI) and ML.
In other words, we are approaching a new era of leadership in federal AI. The introduction of new AI/ML models within federal operations demands greater consideration of the human element within the model lifecycle, as well as the role of applied data science within federal operations. The AI of today and tomorrow must be “responsible AI,” providing data and algorithms that are transparent, equitable, reliable, governable, secure, and resilient.
Opportunities and Challenges
Mission directors are becoming increasingly aware of how data science can:
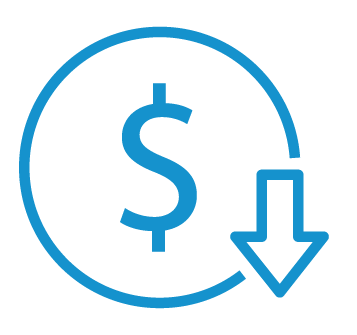
Drive Down Costs
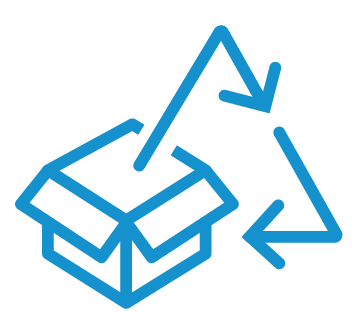
Improve Resource Utilization
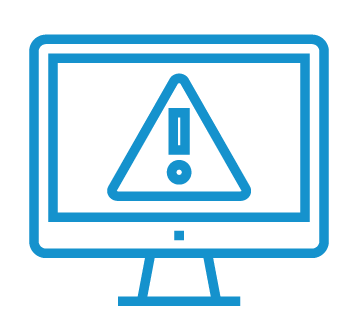
Mitigate Cyber Threats
For example, the National Geospatial-Intelligence Agency (NGA) hosts competitions for the creation of Edge analytics, which empower the warfighter to leverage sophisticated algorithms in disconnected and remote environments. In the same vein, the JAIC has used data science to better measure, model, and engage challenges related to environmental flooding.
Despite this clear appetite, there are several obstacles to the widespread adoption of AI within the federal government. For one thing, commercial startups often fail to understand their federal counterparts—not only their needs, but also their pace and culture, and how to successfully deliver products and services through the federal acquisition process. Moreover, the “work fast and break things” approach of Silicon Valley is ill-suited to federal problems.
In addition, commercial off-the-shelf technology often carries too much risk for government needs. These AI/ML products are either massive tool suites designed for experts or opaque, generalized solutions that do not communicate sufficient trust for federal stakeholders.
Government offices, on the other hand, rarely have enough in-house expertise for data science and algorithm design. Policy-minded federal employees—even those with a strong understanding of their data and the ability to craft scenarios for planning and intervention—are not always well-equipped to transform data through the use of AI/ML.
Bridging The Gap
The best path forward for federal agencies to benefit from ML is the embrace technology to provide robust AI model testing and evaluation. Testing is a state of active research, yet the methods are sufficiently discreet for easy transfer from lab to market, generating a rapid return on federal research investment. While this technology requires an intermediate level of data-science expertise, the sophistication within the labor market is quickly rising to meet this demand.
But the use of AI cannot be limited to technical experts. For trustworthy, tested, and transparent AI to flourish, algorithms must be made more easily understandable for end-users. This is a design challenge that traditional methods like human-centered design cannot solve.
One cannot simply capture user stories to craft a “minimum viable product” for an AI solution. A user-journey map, which demonstrates the benefits of a product on a single user, is insufficient for ML. When deployed at a government scale, ML creates a range of outcomes, which would demand hundreds, if not thousands of user-journey maps. As a consequence, few organizations exist today that can successfully fuse design and AI.
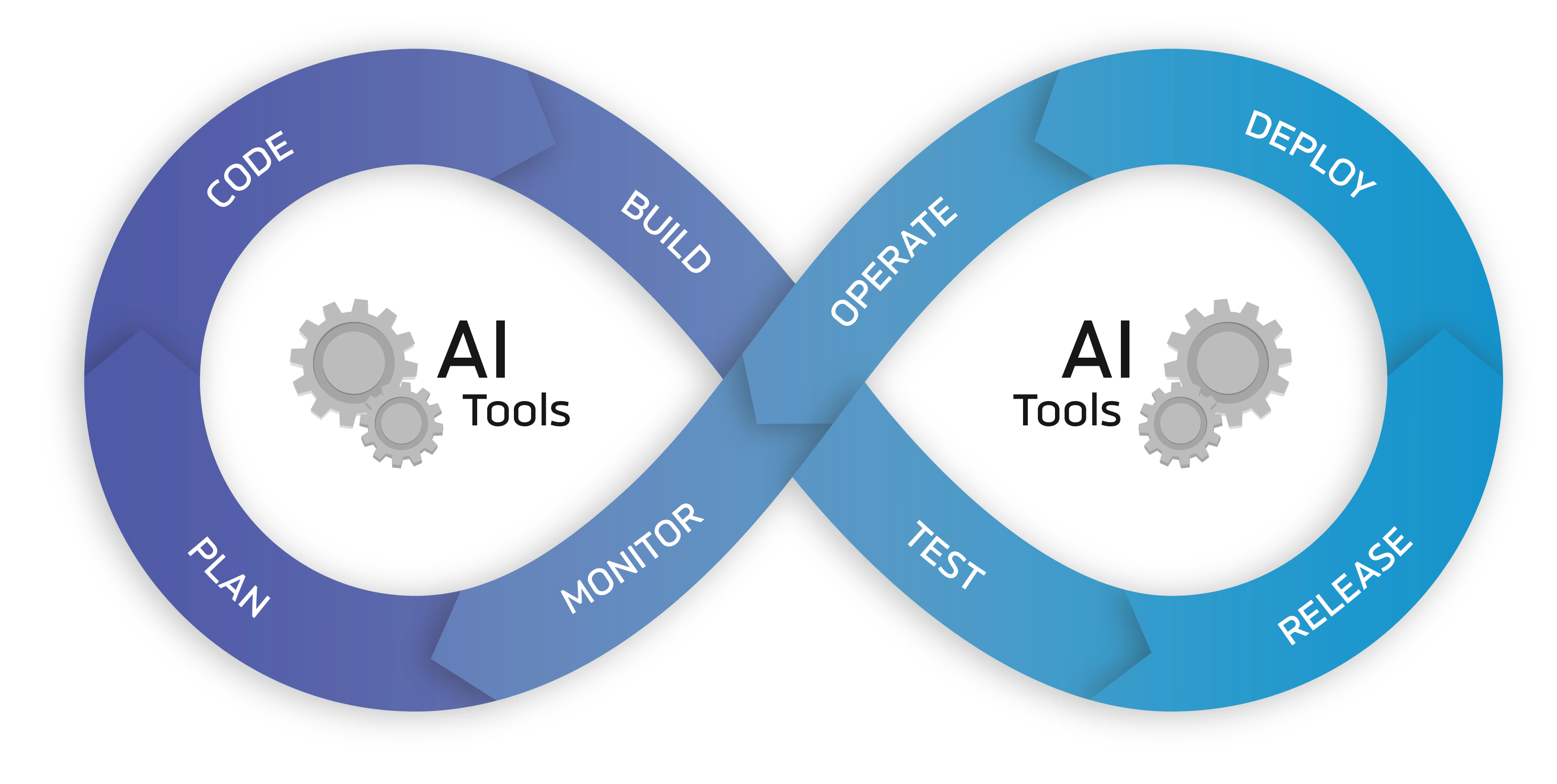
A New Approach
Product is the difference between a roughly hewn capability and a transformative tool. Bad products are obvious, while good products are invisible. In other words, no one notices a hammer unless it’s broken. The same goes for data science—if an AI product is successful, users need not see the algorithms at work, but can simply enjoy the benefits therein. Great algorithms are explainable and easy to use, even though their inner workings may be complex.
In the federal context, good products are also accountable. The flow of data, performance of individual features, and relationship between the algorithm and the problem space must all be made transparent to mitigate problems, inform stakeholders, and aid decision-makers from the operational level to agency leadership and beyond.
At ECS, we build AI solutions that maximize the relationship between the user’s problem, their expertise, and their data to facilitate design-oriented data exploration. We create tools that shift and adapt across user groups, generating benefits to a variety of stakeholders, rather than targeting a single user experience. ECS works with startups like CalypsoAI to experiment with new strategies to bring the greatest value forward.
This approach is vital for the federal domain, where problems and situations change rapidly, and tools must be channeled to benefit large, complex organizations. In today’s increasingly digital environment, command, control, and communication are operational table stakes that accelerate the demand for new capabilities. Only through the use of thoughtful and adaptive applications can we advance these capabilities from certification, collaboration, research, and development through to operational use.
The adoption of data science by the federal government demands a greater consideration of the human experience within AI modeling. Expertise in data science and services is no longer sufficient—thought leadership must extend to products and design. Only with transparent and accountable AI—in other words, responsible AI—will the federal agencies embrace data science and all it has to offer.
Interested in learning more about ECS’ data science and AI solutions?
Reach out and talk to an expert today.