Using Data Mesh to Create a More Efficient and Sustainable Data Ecosystem
Listen to article:
By Patrick Elder,
Director, Data & AI CoE
Anthony Zech,
Director, Data & AI
Ross Serino,
Vice President, Cloud Operations
From Warehouses to Lakes to Swamps: How Did Data Architecture Get Here?
Decades ago, when maturing organizations began to recognize a need for systems that could manage and analyze large volumes of data, the first data warehouses were born. This enabled centralized, standardized reporting and analysis, but over time it became clear that making changes in data warehouses was too slow to keep up with the mission’s pace. What followed? Data lakes, unstructured repositories that data flows into without transformation, enabling centralized teams to take on the data preparation load for rapidly evolving analytic needs.
Unfortunately, data lakes tend towards disorganization over time, which is where the term “data swamp” came from. Much like a swamp’s murky waters, the ungoverned mixing of data sources and types makes it increasingly difficult for analysts to navigate, especially at mission speed.
The need for clarity, efficiency, and sustainability brings us to the next step in the evolution of your data architecture: a distributed model, a.k.a. data mesh.
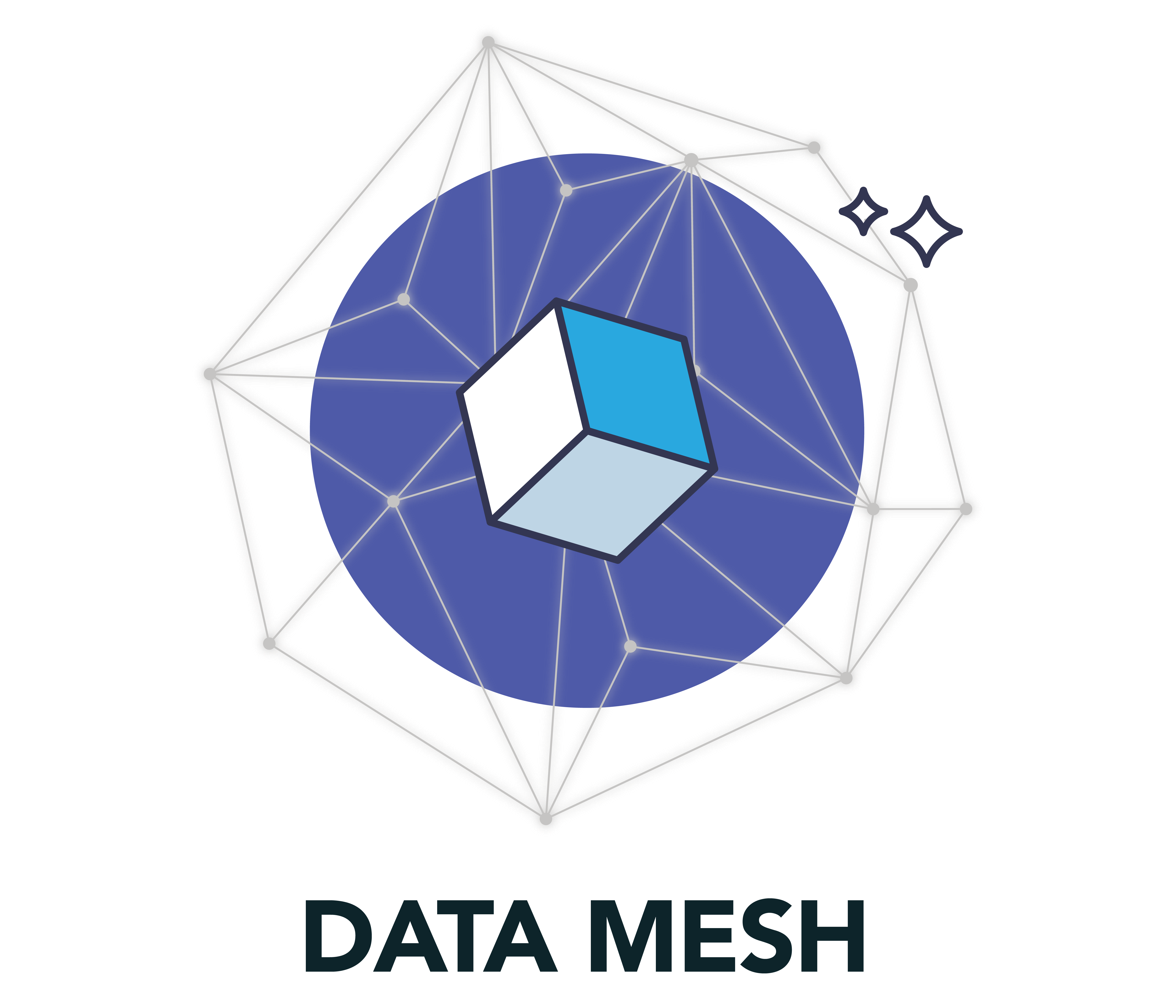
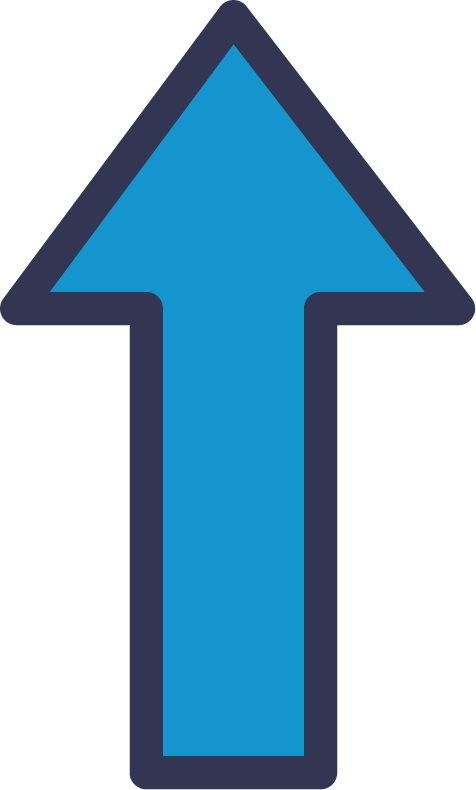
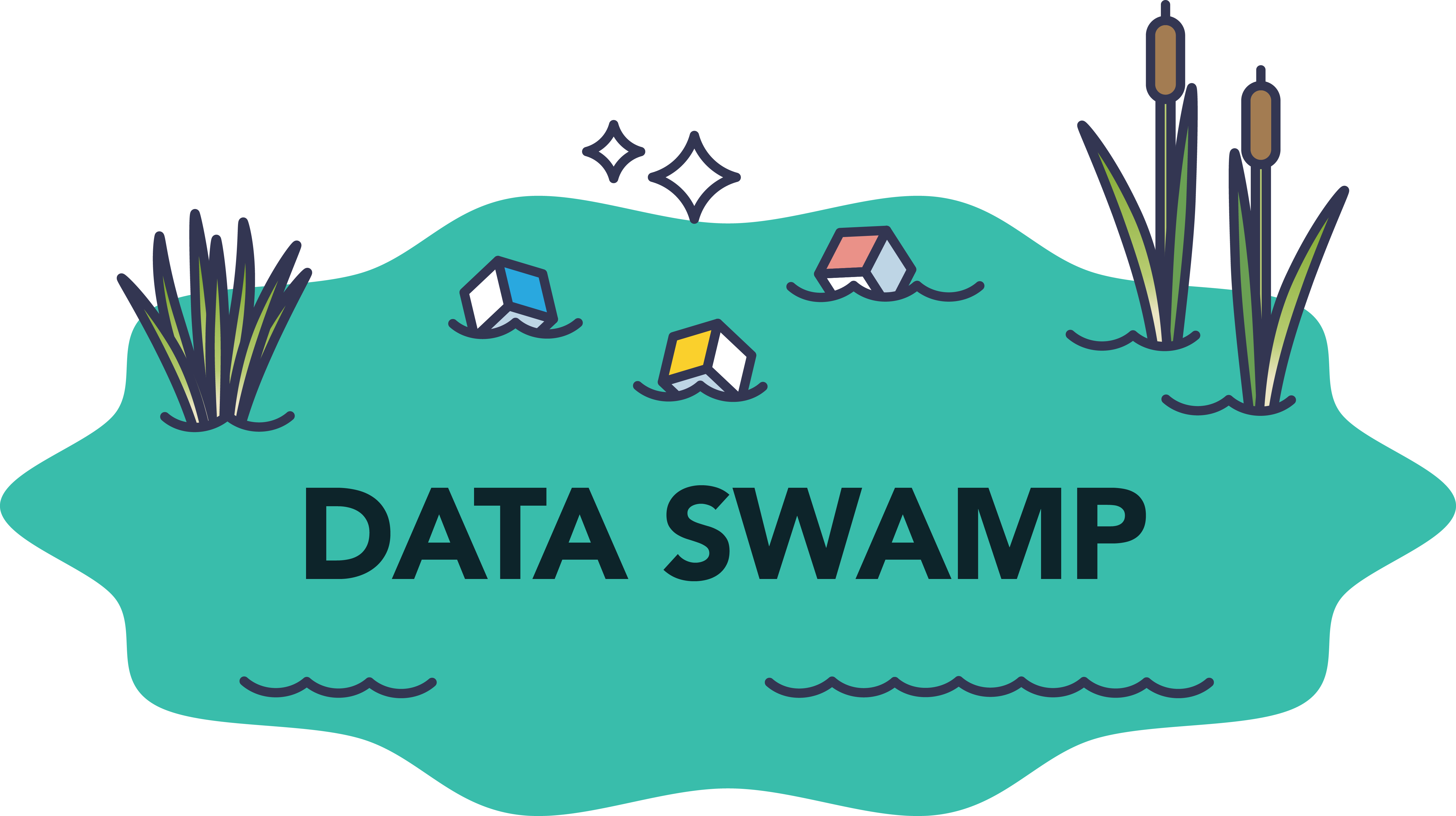
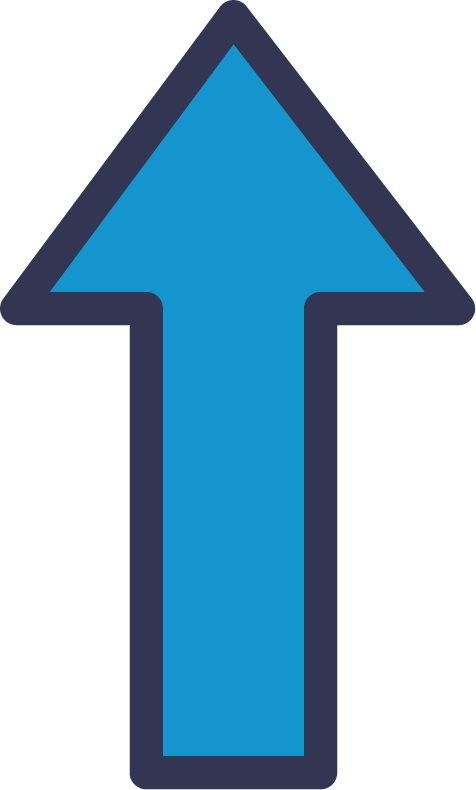
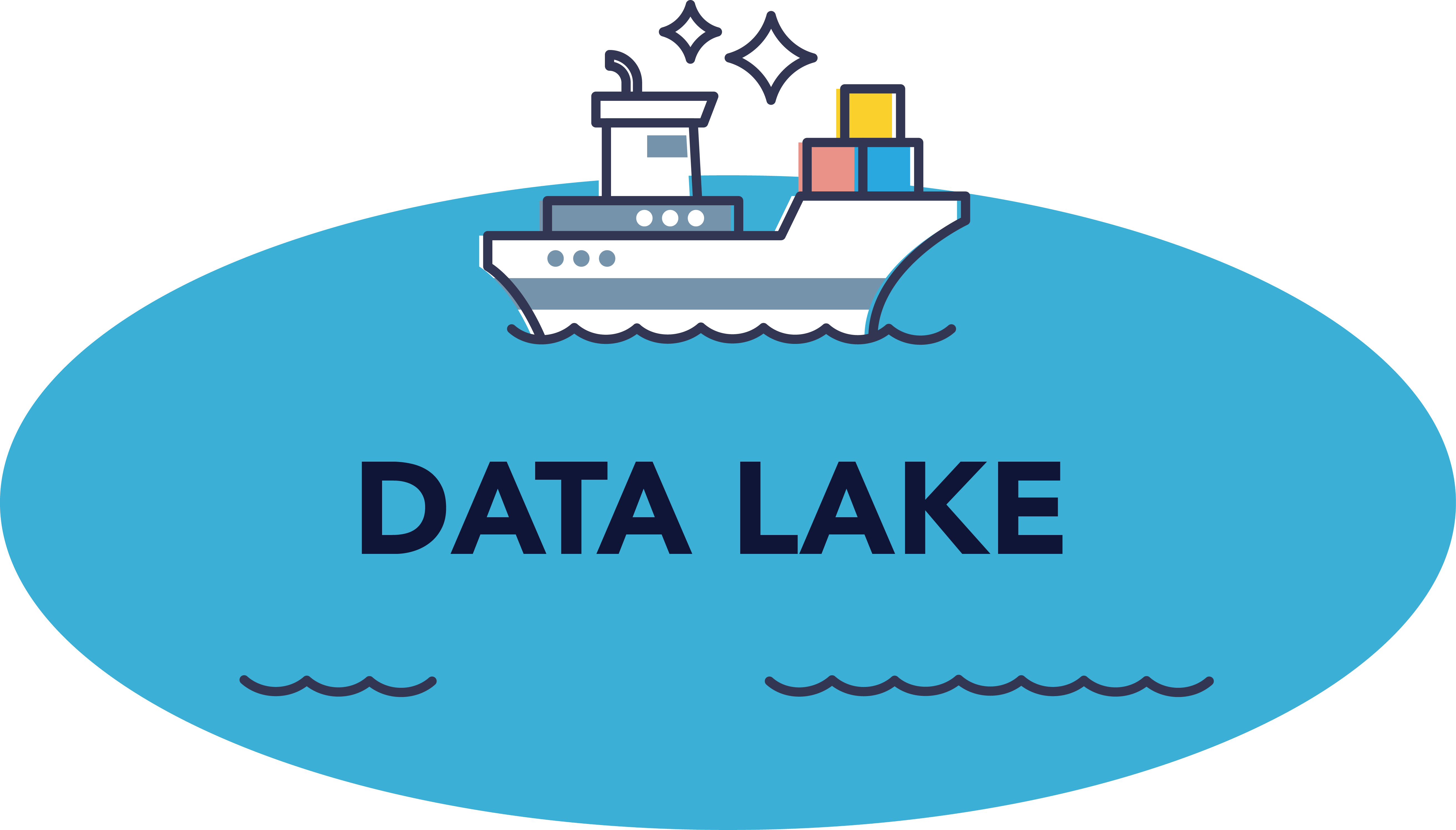
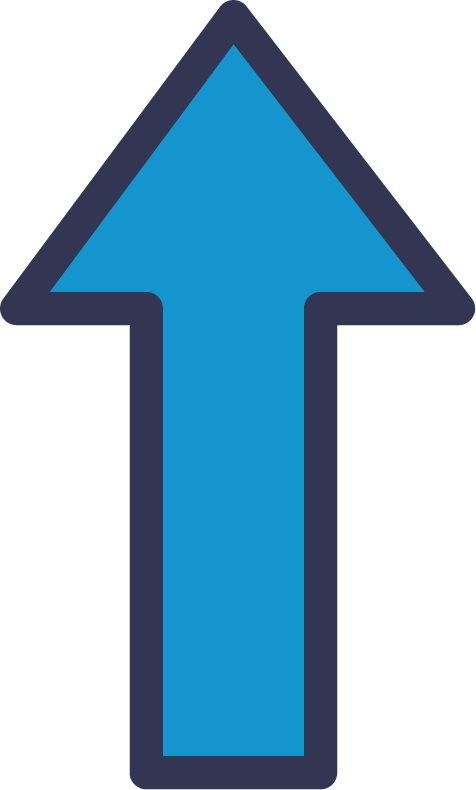
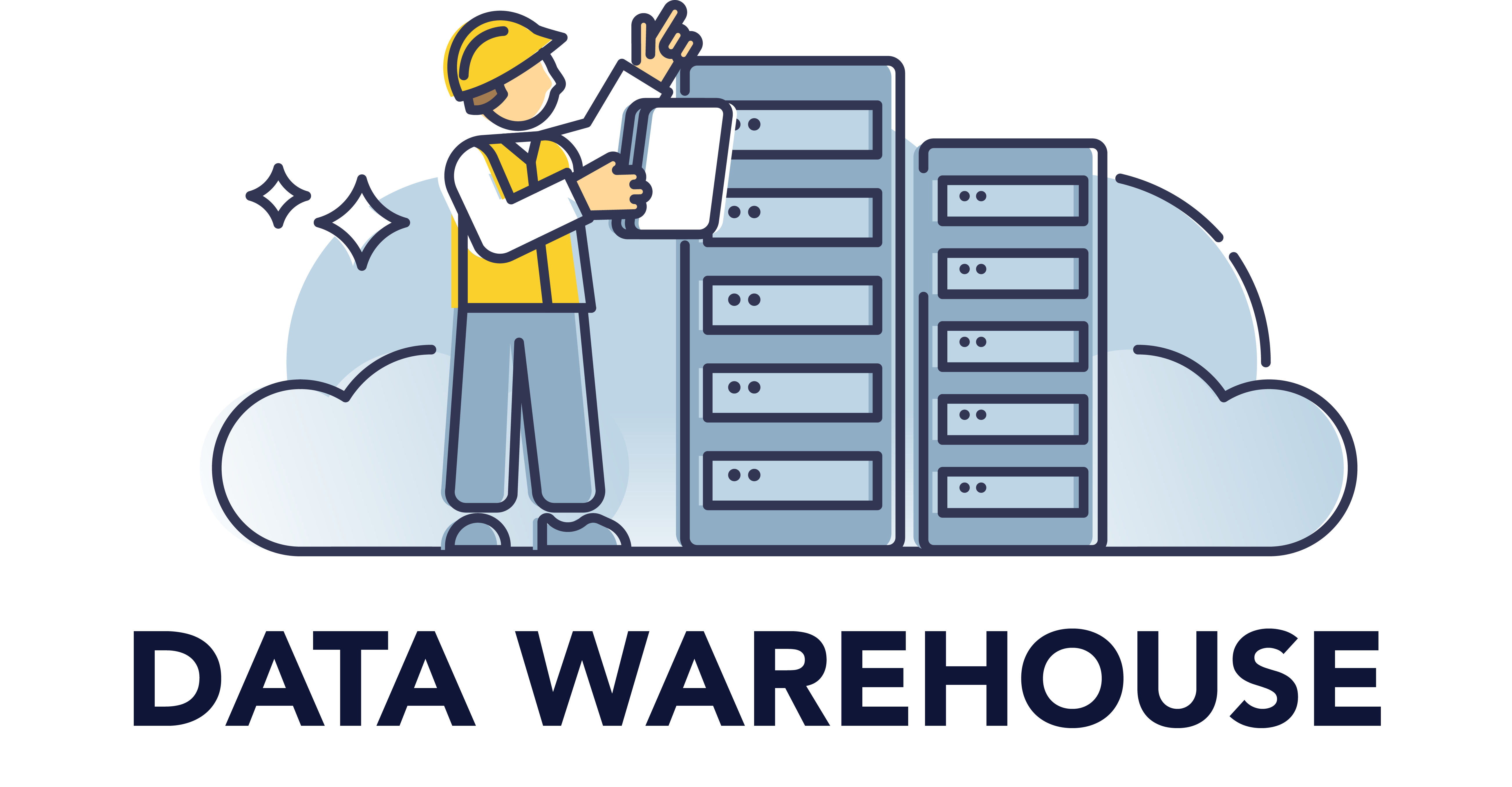
Data mesh was embraced by the U.S. Army in the October 2022 Army Data Plan
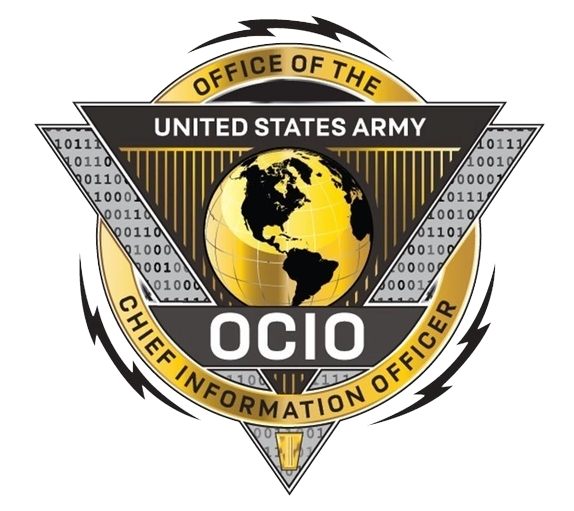
Escaping the Data Swamp
WithData Mesh
Data mesh architecture’s distributed model of data management represents a leap forward in organizational maturity. By decentralizing data ownership to domain experts focused on the creation and maintenance of data products, then making those products discoverable within a centralized, curated data catalog, data mesh transforms the way your organization handles and utilizes data.
Here are the three essential ways that data mesh improves upon traditional
data architecture and helps your organization escape the data swamp:
1. Decentralized Ownership and Federated Governance
What It Is: Centralized data architecture creates bottlenecks, scalability challenges, and a lack of agility. In contrast, data mesh embraces decentralization, which fosters a more dynamic and scalable approach to data management, and federated governance, which enables seamless integration and collaboration across different parts of an organization.
The Advantages: One of the key advantages of decentralized ownership lies in creating a direct line of communication between data users and data owners/producers, allowing the mission needs of the former to inform the work of the latter. As data owners focus on the creation of valuable data products for the data catalog, users can weigh in with what exactly they’re looking for, enhancing products’ usefulness. This collaborative approach stands in direct contrast to the imposing, “hunt and find” nature of a data swamp.
Learn how Data Mesh architecture can help your organization meet the standards of the Federal Data Strategy
2. Domain-driven Data Products
What It Is: At the heart of data mesh is the idea of treating data as a product. This means each data set is carefully curated, maintained, and served by a domain-specific team that understands its context, use cases, and users. By doing so, data products become more relevant, reliable, and accessible to those who need them, transforming data into an asset that drives decision-making and innovation.
The Advantages: Data mesh’s focus on local expertise and autonomy leads to better quality data products that are closely aligned with team objectives. By reducing dependency on central data teams, data mesh enables quicker access to data and faster time to insights while enabling teams to iterate rapidly. It also leads to higher quality analysis because users know exactly what they are getting from a data product, reducing the risk of incorrect assumptions or interpretations that can lead to poor decision making.
3. Observability and Data Integrity
What It Is: In data mesh architecture, observability — visibility into the operational health of your data infrastructure — becomes an inherent feature at every level of the data ecosystem. Observability equates to the ability to proactively manage and oversee the health, quality, and performance of data pipelines, processes, and systems.
The Advantages: Observability provides a clear, auditable trail of how data is accessed, used, and transformed, improving governance and compliance and building trust in the data and the insights derived from it. Ultimately, observability helps ensure data quality and integrity while also boosting operational efficiency, as clarity around the state of your data infrastructure can help reduce downtime and smooth out processes.
Maturing Your Data Architecture
Data mesh offers many significant advantages over traditional data architecture and allows you to escape your data swamps before they can negatively impact your productivity, decision making, or regulatory compliance.
However, implementing data mesh comes with its own challenges. Your organization must:

Determine your domains of expertise and assign data product owners who will manage their data products from end to end. There should be a structure in place to resolve any disputes across domains that may arise.

Develop the necessary infrastructure to support a distributed architecture, including data pipelines, storage solutions, and governance mechanisms that can work across various domains. Implementing a centralized data catalog where data products can be easily discovered is critical to making data mesh architecture work.

Cultivate a culture where data is valued as a product, which will require training, incentivizing, or even restructuring teams to embrace this new mindset.
You will also inevitably face issues around standardization, data security, and the complexity of managing multiple systems, all of which should be addressed at the appropriate domain level.
One proven method to navigate these challenges and revolutionize your data architecture is partnering with our experts at ECS. We are committed to helping federal organizations complete the transformation from traditional data architecture to data mesh, escape their data swamps, and create more efficient, sustainable data ecosystems.